Selpi Selpi
My current research interests are on data-centric AI, learning and decision making from multiple sensors (multimodal and multi-view learning), unsupervised learning, interpretable AI, and their applications to e.g., autonomous driving and traffic safety. Check out this link to read a bit of my work in the EU project called PANACEA, and watch this podcast to get some updated information.
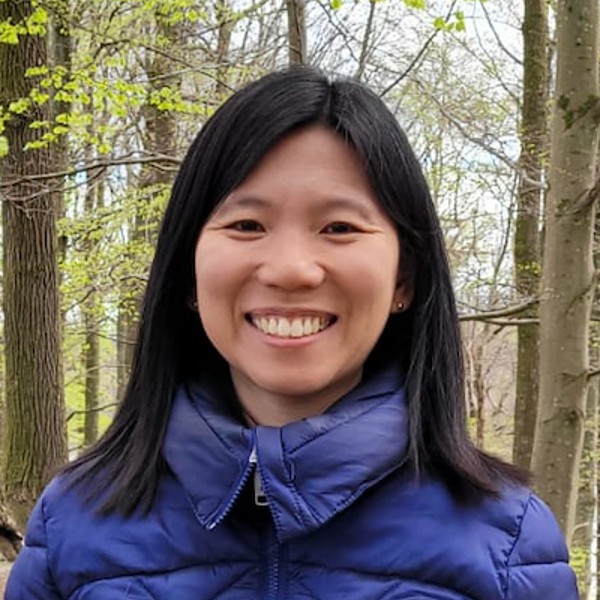
Showing 38 publications
Spatiotemporal Interaction Pattern Recognition and Risk Evolution Analysis During Lane Changes
Potential impact of autonomous vehicles in mixed traffic from simulation using real traffic flow
Cooperative merging strategy between connected autonomous vehicles in mixed traffic
Optimization of Two-Phase Sampling Designs with Application to Naturalistic Driving Studies
Behavioral adaptation of drivers when driving among automated vehicles
Safety benefit of cooperative control for heterogeneous traffic on-ramp merging
Analytical Method of Traffic Conflict at Urban Road Intersections Based on Risk Region
Lane-Level Map Matching based on HMM
Simulation-based impact projection of autonomous vehicle deployment using real traffic flow
Comparison of Car-Following Behavior in Terms of Safety Indicators Between China and Sweden
Safety-centred analysis of transition stages to traffic with fully autonomous vehicles
Characterisation of Motorway Driving Style Using Naturalistic Driving Data
Analysis of SHRP2 Data to Understand Normal and Abnormal Driving Behavior in Work Zones
The Relationship between Different Safety Indicators in Car-following Situations
Economics of Road Safety – What does it imply under the 2030 Agenda for Sustainable Development?
A Review of Research on Driving Styles and Road Safety
The effect of curve geometry on driver behaviour in curves by using naturalistic driving data
Mutual Recognition Methodology Development
Deliverable D5.3: Final delivery of data and answers to questionnaires
Deliverable D11.3: Final Report
Deliverable D3.3: Data management in euroFOT
Automatic real-time FACS-coder to anonymise drivers in eye tracker videos
On data security and analysis platforms for analysis of naturalistic driving data
Predicting functional upstream open reading frames in Saccharomyces cerevisiae
Using mRNA Secondary Structure Predictions Improves Recognition of Known Yeast Functional uORFs
Using Inductive Logic Programming to Predict Functional Upstream Open Reading Frames in Yeast
A First Step towards Learning which uORFs Regulate Gene Expression
Using the Functional Data Model to Store and Query Recursive Biological Data
Pathway and Protein Interaction Data: from XML to FDM Database
Download publication list
You can download this list to your computer.
Filter and download publication list
As logged in user (Chalmers employee) you find more export functions in MyResearch.
You may also import these directly to Zotero or Mendeley by using a browser plugin. These are found herer:
Zotero Connector
Mendeley Web Importer
The service SwePub offers export of contents from Research in other formats, such as Harvard and Oxford in .RIS, BibTex and RefWorks format.
Showing 16 research projects
An interpretable, self-explaining method for traffic safety applications
Deep MultiModal Learning for Automotive Applications
PracticAl and Effective tools to moNitor and Assess CommErciAl drivers’ fitness to drive (PANACEA)
Personal mobility and development
Aligning ontologies in traffic safety and urban development
Heterogeneous Traffic Groups Cooperative Driving Behaviours Research under Mixed Traffic Condition
Driving styles of autonomous vehicles in mixed traffic (DS-Auto)
Public transit shared mobility - connected and safe solutions
Creating a core-enabler for evaluating scenarios of mixed vehicular traffic
Analysis of SHRP2 Data to Understand Normal and Abnormal Driving Behavior in Work Zones: Phase II
Analysis of SHRP2 Data to Understand Normal and Abnormal Driving Behavior in Work Zones: Phase I
Att bättre förstå körstil från naturalistisk kördata
Driver modelling and cross-cultural analysis of driving styles based on large-scale driving data